In the era marked by sensors and interconnected devices, data scientists encounter new challenges. Their primary obstacle lies in efficiently ingesting and utilizing both homogeneous and heterogeneous IoT data sourced from various sensor devices. With the business landscape witnessing the emergence of new devices with diverse sensor parameters, necessitating continuous monitoring for optimal operations.
Big Data Challenges
Managing homogeneous and heterogeneous data ingestion and utilization stands as just one of the challenges confronting IoT practitioners. Additional challenges in data volume storage include the following.
Data Volume Management: Balancing the imperative to retain all big data from IoT devices to prevent data loss against the need to avoid allocating resources to unnecessary data that might not require analysis.
Omnichannel Source Data: Encountering complexities in capturing data from multiple sources due to variations in device architectures. For instance, legacy devices may store data in one file format on a relational database, while newer devices may adopt different formats, necessitating comprehensive data management to leverage all available data.
Heterogeneous Data: Dealing with diverse smart sensor devices that may report data with the same parameters but in different units due to varying reporting and measuring standards. This heterogeneity poses challenges for reporting, requiring conversion into a standardized reporting metric.
The Solution: SAP HANA
To address the imperative of capturing sensor data without loss and enabling its efficient storage and analysis, a robust big data platform is essential. SAP HANA's in-memory database offers a solution that fulfils both requirements. It serves as an optimized storage mechanism for IoT data and facilitates real-time device monitoring, all while remaining cost-effective for businesses.
Moving data to SAP HANA's memory layer allows continuous data storage without indexing, enabling the insertion of data across multiple partitions without blocking write operations. The partitioning is customized based on data quality and type obtained from the streaming layer.
Benefits of Clean Big Data
Importing clean data into SAP HANA yields several advantages. Firstly, cleansed data ensures maximum utilization and value. Seamless management of omnichannel data enables successful analysis and predictive modeling of device outputs. Also, a clean and well-maintained database aids enterprises in effectively utilizing sensors and leveraging existing data to predict device failures or maintenance needs.
By employing compression techniques like Apache Hadoop's parquet formatting, which utilizes the snappy algorithm to compress data, data homogenization is achieved, reducing input-output overhead. Storing data of the same type in each column in binary format enables the utilization of encoding optimized for modern processors, enhancing instruction branching predictability.
Efficient storage of IoT data is important for leveraging insights from sensor devices. Gemini Consulting & Services can help you leverage SAP HANA to handle IoT data. To find solutions to the challenges encountered by database administrators in handling IoT data and using cost-effective storage solutions like SAP HANA and SAP Vora, Contact us.
In addressing big data challenges, SAP HANA collaborates with both SAP and non-SAP tools, offering a comprehensive solution. Let's explore its functionalities.
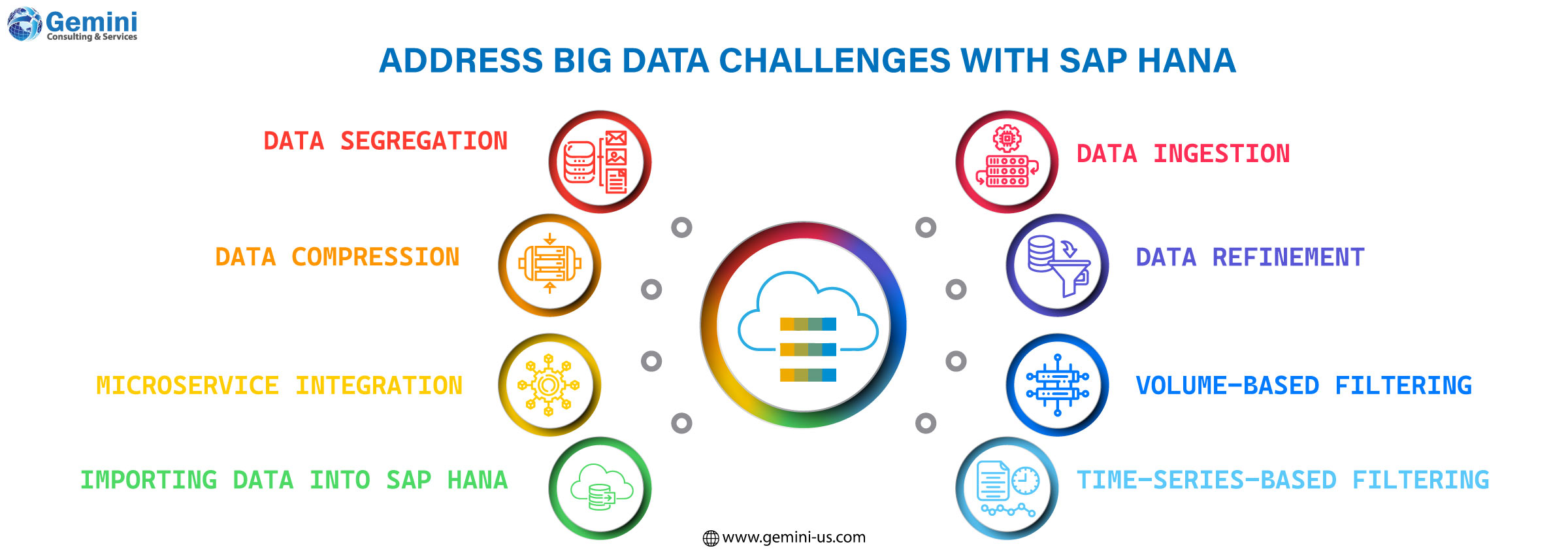
Data Segregation
SAP HANA's architecture enables the creation of multiple partitions, facilitating efficient management of big data. Administrators can categorize data into these partitions based on various parameters such as sensor types, geographic regions, or operational units.
Data Compression
Efficient data compression is crucial for minimizing storage requirements. Familiar to Apache Hadoop, users can format like parquet and Optimized Row Columnar (ORC), which significantly reduce data footprint. SAP HANA integrates with tools like SAP Vora to store data in the compressed parquet format, enhancing query performance by reducing data scanning overhead.
Microservice Integration
Prior to data storage in SAP HANA, eliminating redundant data is advisable. Leveraging Spring Boot applications enables parallel processing, further reducing the burden on SAP HANA databases.
Importing Data into SAP HANA
Let's delve into the process of importing data into an SAP HANA database, particularly focusing on IoT data storage.
Data Ingestion
The initial step involves ingesting raw IoT data into a landing container within the SAP HANA database. During this stage, indexing is deferred to expedite storage, prioritizing the swift accumulation of raw data.
Data Refinement
Subsequently, attention shifts towards refining and compressing the data to optimize storage and analysis within SAP HANA's memory layer. Various filtering methods are employed for this purpose.
Volume-Based Filtering
Data volume filtering scrutinizes the sizes of individual data packets, excluding those that deviate from predefined size thresholds. This approach aids in identifying anomalies such as malfunctioning sensors or signal loss.
Time-Series-Based Filtering
In time-related data analysis, a time-series filter evaluates standard deviation and timeliness to streamline sensor data presentation. This method effectively reduces redundant data instances, resulting in significant storage savings.
By employing these strategies, SAP HANA optimizes data management and analysis, offering a robust solution to complex big data challenges.
Synchronize, Store and Access Data
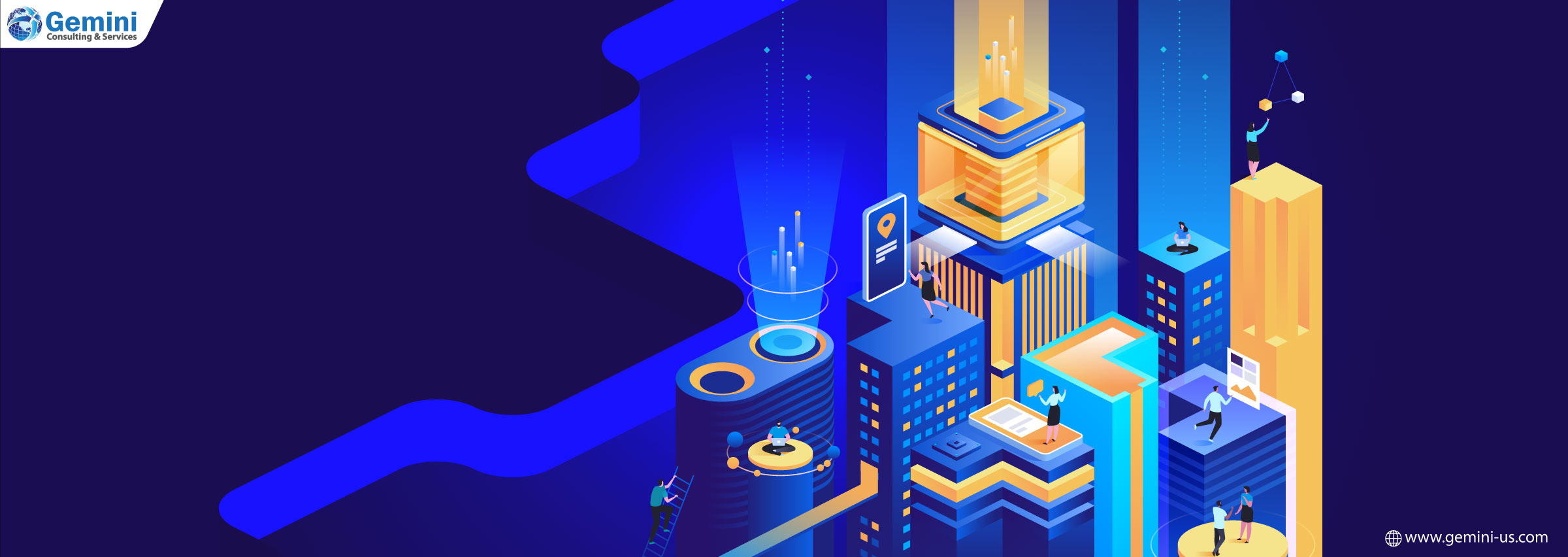
To compress the data effectively, we must convert the cleansed data into a binary format suitable for querying. Apache Hadoop offers solutions to compress data into formats like ORC or Parquet. Once this conversion is complete, the data will be primed for loading into an SAP HANA database.
To manage a substantial influx of data seamlessly and ensure none is lost, it's imperative to establish a microservice or isolation layer between the incoming data destined for the SAP HANA database and the existing IoT data. These microservices can be developed using various languages such as Python, Java, Groovy, or Kotlin, and can adopt industrial formats like AVRO, Parquet, ORC, JSON, CRV, among others. This layer will handle incoming data, orchestrating batch operations to insert data into the memory layer of the SAP HANA database.
Storing data in the memory layer enables continuous data storage without the need for indexing and facilitates the utilization of multiple partitions. This allows data insertion without any need for changing write operations. Partitioning strategies should be devised based on data quality and type received from the streaming layer.
With your data now stored in the SAP HANA database, users can access it seamlessly. Since relevant and cleansed data resides in memory, real-time information retrieval becomes possible. Additionally, data no longer required can be routinely purged from this state and shifted down a layer, freeing up memory space for subsequent analyses.